AI off the Rails
15 April 2022
CDH Faculty Director Meredith Martin responds to Emily M. Bender’s presentation on the paper “AI and the Everything in the Whole Wide World Benchmark” at recent Rutgers-ANU Data Ontologies workshop.

This post is a reflection on the third Data Ontologies workshop, the second in a two-part series of AY 2021-22 workshops organized through a Rutgers Global and NEH-supported collaboration between Critical AI@Rutgers and the Australian National University. Click here for the workshop video and the discussion that followed. This blog post was originally posted on the Critical AI blog.
Emily M. Bender’s March 24 presentation for the Rutgers-ANU DATA ONTOLOGIES workshop centered on the co-authored paper: “AI and the Everything in the Whole Wide World Benchmark.” Bender’s talk was a clarifying complement to the paper, ideally suited to spark an interdisciplinary discussion.
To begin with, what is a benchmark? Raji, Bender et. al. write “In this paper we describe a benchmark as a particular combination of a dataset or sets of datasets (at least test data, sometimes also training data), and a metric, conceptualized as representing one or more specific tasks or sets of abilities” (2). So a benchmark is dataset(s)+task. Following the paper, Bender’s talk explored how the intense competition to train models that perform efficiently based on such benchmarks normalizes the metrics that benchmarks embed while ignoring the dangers and limitations that attend such uncritical focus. As such, benchmarks encourage researchers to measure against a supposedly “general” marker of successful research that, in actuality, may lack “construct validity”--that is, may lack the ability to measure what it claims to.
At the highest level, Bender criticized the profession and industry for, in effect, rewarding speedy and often superficial progress over robust and time-consuming research. The practice of “SOTA (state-of-the art) chasing” incentivizes shallow measures of performance without regard for the value or application of these supposed advances. Arguing instead for a slow and careful science that brings scholars together–likely from different disciplines–who are the most qualified to assess the appropriateness of a benchmark for answering a research question, Bender challenged the conventional notion that the field of machine learning is accelerating scholarly discovery. Instead, what became clearer in her talk is that “benchmarks” that profess to measure generalizability–that is, progress toward the goal of a human-like “artificial general intelligence” (AGI)--are inaccurate and dangerous. Natural Language Processing (NLP) and Computer Vision (CV) benchmarks should be specific–tuned to a particular dataset and task. Because popular benchmark competitions imply that the datasets on which they are based are general enough to serve as representative, the lie of “generality,” as Bender showed, is itself a central problem in the pursuit of, and hype around, AGI.
Bender took a very humanistic approach to the concept of “benchmarking,” referencing the history of the term more centrally in her presentation than in the paper (where benchmark as a term is explored in the appendix). This move acknowledged the historical embeddedness and contextual specificity of the terms we use to describe our research and, in that way, reminded me of work by media theorists Shannon Mattern and John Durham Peters. By using the metaphor of “everything in the whole wide world,” borrowed from a 1974 Sesame Street children’s book, Bender could reach an interdisciplinary audience to emphasize the importance of approaching categories with a critical eye. Literary critics might be tempted to invoke the work of Jacques Derrida or Michel Foucault to describe the power or inadequacy of language; book historians and information theorists might refer to the important history of categorization and its consequences. Nonetheless, Grover is not only familiar to an interdisciplinary audience but also nods to the trend of using Jim Henson’s creations in AI.
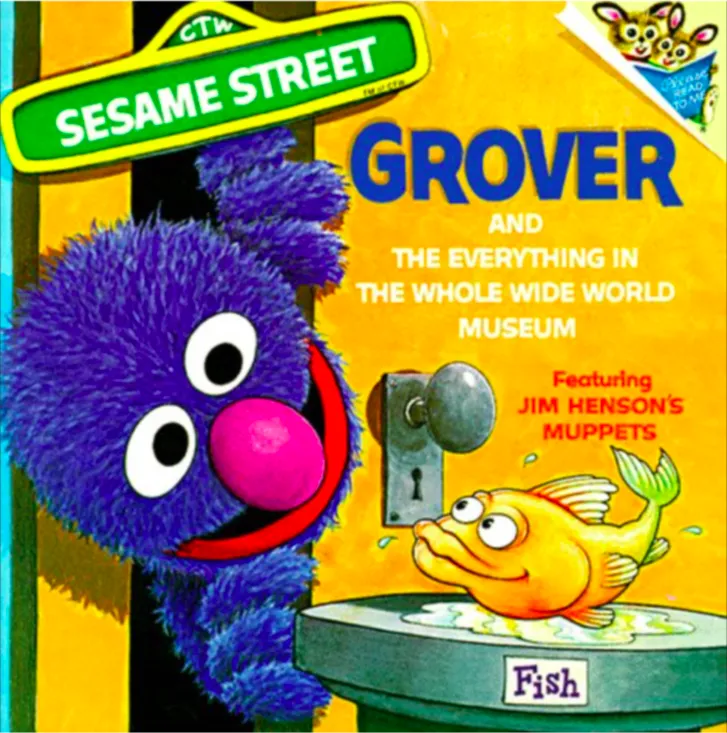
For those who haven’t read the article or the children’s book, Grover is exploring a museum that claims to showcase everything in the world, but the exhibit categories are arbitrary and incomplete, and only “include” everything else in the world by way of a door to the outside. With Grover as a friendly guide, Raji, Bender and colleagues show how similarly misnamed “general” benchmarks cannot possibly “include” everything that would make them “general” to begin with. The unexamined and arbitrary categorization in Grover’s museum parallels the ways that the benchmark datasets (such as ImageNet or WordNet) are often mismatched with the tasks that machine learning researchers (and those who fund them) have been pushing to “solve”. That is to say, the museum categories lack “construct validity.” By wrongly assuming that benchmarks are general, researchers claim that advances in NLP and CV have made major progress toward “general-purpose capabilities” (Raji et al. 1).
As an example of the arbitrariness that plagues the field’s research culture, Raji, Bender et al. point to Kiri Wagstaff’s remarks on the accuracy of iris and mushroom classifiers which have had no impact for researchers in fields such as botany or mycology. Rather, the research appears to have been inspired by the existence of a tractable dataset–not a research problem that specialists hoped to solve. If machine-driven image classification is a hammer, then these datasets are a readily available supply of nails that can be hit with increasing force and accuracy — even if those nails aren’t actually holding anything together or building anything up.
As a field, “AI” refers to many domains; but the generalizability at stake for those building these systems is the decades-old goal of AGI: that is, systems that can flexibly solve a range of problems in the way that humans do when they transfer knowledge about and experiences in the world from one task to another. This would turn “AI” (which can refer to a machine very good at playing chess but not good at much else) into “AGI (a machine that while good at playing chess could successfully solve a different kind of puzzle, or, even more unlikely, write a poem).
Of course, knowledge is different from information, as media and literary theorists know quite well. If the goal of much AI research is to design a set of pragmatic models “that can generalize (with minimal fine-tuning) to a wide range of other tasks they were not specifically developed for'' (2), then it makes sense to train models on language or images. What better “data'' for generating something humans are already trained to read as meaningful (even if they are not media or literary theorists). And yet, claims about AI’s supposed successes in generalizing the “whole wide world” ignore the continuing narrowness and rigidity of AI systems–both in the constructed nature of their datasets and in the mismatched tasks that are assigned by these systems. Moreover, these hyped achievements continue to cause real harm, and to jeopardize what actual scientific discovery might be realized through robust study of language and image.
Talking Across Disciplines
It’s been about three weeks since Bender’s talk and I’ve been sitting with her ideas. I am grateful for her work and for the work of Bender, Timnit Gebru, Margaret Mitchell, and Angelina McMillan-Major. Their paper, “On the Dangers of Stochastic Parrots: Can Language Models Be Too Big?” as well as Gebru et. al’s “Datasheets for Datasets” have provided a way into these conversations for me and others at the Center for Digital Humanities at Princeton which is devoted to the study of humanities data and the broadly interdisciplinary conversations Bender and others have been starting since our “Year of Data” in 2018, which launched Ruha Benjamin’s Ida B. Wells Just Data Lab and welcomed Safiya Noble as its keynote. Inspired largely by the Bender “rule,” our associate director won an NEH Grant to design workflows to help scholars create data and train models for languages that are currently unsupported by NLP tools. We read and discussed this research in our humanities + machine learning working group and we hosted an event last October that brought together leading digital humanities scholars with two of the co-authors of “Stochastic Parrots” (the position papers from that event will be published soon as issue 3 of our in-house journal Startwords and the paper was also discussed during a proximate Critical AI event and blog).
What has troubled me since Bender’s talk (and another fantastic elaboration of the paper by Emily Denton at Princeton’s Center for Information and Technology Policy on April 5th) was not the talk itself, but an unsettled feeling – similar to the way I felt after the conversation last October (which also included Ted Underwood, Lauren Klein, Gimena del Rio Riande). There are too many disparate conversations happening without anything that could inspire a collective response for those of us who work in the humanities and the arts, those who work in linguistics (I’m not even going to get into that tangle), those who work in the computational social sciences, and those who work in machine learning and computer science — in universities, but even more crucially, in industry.
In the fall discussion, it seemed as if the representatives of my own field, roughly comprising literary criticism and cultural studies, had no unified approach to the problem of large language models. These large language models (LLMs) were controversially anointed as “foundation” models by Stanford only six months after “Stochastic Parrots” raised the alarm about the harm they were causing; so though the terms might appear interchangeable to some audiences, not everyone agrees that LLMs should be considered “foundational” at all (a topic Meg brought up in our October discussion). Ted’s perspective was positive: excitement at the ways literary and cultural critics would be able to interpret new creative outputs–especially artistic–based on large language and image models. For Ted, the train has left the station and the best way for a humanist to be involved is (as I understand him) to describe and interpret and wonder at the brave new world that has such strange and interesting machine-generated text and images in it. Lauren’s perspective was much more cautious; if we continue with the train metaphor, we can imagine Lauren saying, “let’s please blow up the tracks, build a new infrastructure, and maybe rethink train travel.” Gimena sounded a warning from a different perspective, reminding us that there are entire continents that aren’t even allowed to get on the train, and are therefore being left behind. Meg and Angelina were certain that having humanists in the room–scholars with a wide array of theoretical, historical, and literary expertise and knowledge–would help to make the data more accurate and fair. They seemed to think it possible to conceive a train trip on which everybody was invited. The positive hope for the future that Meg wished for was one in which the models didn’t cause harm. In fact, Meg was taken aback at Ted’s positive-seeming spin. Yes, the train has left the station, she seemed to say, but that means that the models are being deployed and, thus, causing harm.
What can we do to catch this speeding train? Can we? This metaphor has gone off the rails, but in our fall conversation as well as in that which followed Bender’s Critical AI presentation, everyone seemed to agree that real harm was and is being done, and that such harm outweighed the perceived benefits of these large language models.
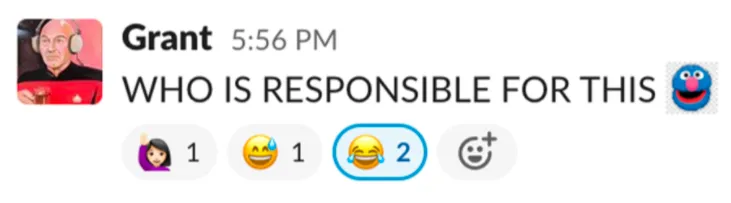
So what bothers me the most – and what Bender was keenly attuned to – is that even as misleading benchmarks facilitate hype–and even as hype results in inaccurate and harmful claims about culture– humanists, social scientists, computational linguists, and sociolinguists don’t have a common understanding, or even common language, through which to launch a unified response. That is to say, when over-generalizing claims about benchmarks that are less generalizable than they purport to be hide the harm, (or make it seem as if the harm is a necessary byproduct of progress), we can each protest in our own fields, but we don’t yet have a way to talk broadly in academia (and still less industry) about how we might collaborate toward reversing these trends.
By showing that not only the claims, but also the benchmarks are misguided, Raji, Bender et.al. urge us to think about how we represent and portray machine learning to the general public. They are also asking us to educate ourselves so that we don’t buy the hype. Not surprisingly, this tendency is rampant from writers covering industry’s supposed “innovation,” and even from researchers themselves (including researchers in industry). It’s not only that claims of generality fuel AI hype (using phrases to describe models that “beat human performance on a broad range of language understanding tasks” or have the “ability to handle the complexity of the real world”) but they also ignore what Bender’s co-written research showed again and again: “The imagined artifact of the ‘general’ benchmark does not actually exist. Real data is designed, subjective, and limited in ways that necessitate a different framing from that of any claim to general knowledge or general-purpose capabilities” (5). As researchers chase after faster and ostensibly more impressive results, the benchmarks they use to make their claims are not sufficiently questioned.
Though we can all unify about the concept of “harm” fairly easily and effectively, perhaps we might also begin to talk in a more unified way about the adjacent concept of “data.”
Johanna Drucker introduced the concept of “Capta'' back in 2011 to distinguish between data (considered as objective “information”) and capta (to highlight information that is captured because it conforms to the rules and hypothesis set for a given experiment). In “Humanities Approaches to Graphical Display” (2011) and Graphesis (2014) Drucker’s re-orientation toward the interpretation and situatedness of data, in concert with Lisa Gitelman’s edited collection Raw Data is an Oxymoron (2013), influenced the strand of “digital humanities” that works in critical data studies.
Institutionalized DH over the past decade suffered when it was associated primarily with tools and accelerated discovery at the expense of the use-value of good research questions, theory and critique (not across the board, but in the most hyped examples). But series such as CDH’s Year of Data in 2018-2019 and the ongoing Rutgers-ANU workshops (co-organized by DH scholar Katherine Bode) have brought interdisciplinary discussions of data science, the ethics of data curation, and data ontologies to large audiences. There is no lack of current discussion about the state of critical data studies, book history, and critical archival studies in digital humanities; DH scholars have been theorizing about this topic for the same amount of time that DH has been fielding critiques about the perception of outsized institutional support. We all know that the best, most lasting impact of DH as a field (if we think of it that way) is its theoretical, methodological, and critical orientation. The recent, more public turn in DH toward an understanding of harm and its possible correctives via Lauren Klein and Catherine D’Ignazio’s widely adopted Data Feminism doesn’t mean that we can’t keep exploring distant horizons of literary scholarship. But it does mean that we need to move deliberately and slowly as we do so, bearing in mind how our data is always situated (including the interpretive work necessary for “cleaning” data or making it “tractable” in other ways), and how our methods are only as strong as the research questions that necessitate them. Moreover, as we undertake this deliberate and critical work, we need to think of its impact as equal to, if not more important than, the impact of faster, yet often faultier, performance. Again, this is not new in the field of DH, but until Klein and D’Ignazio’s book we hadn’t done the best job translating our methodologies to the larger data science community.
It’s not just humanists who have been re-orienting how we think about data – social scientists like Duncan Watts (2004) and Matt Salganik have had profound effects in Computational Social Science, recognizing the need to bring contextual knowledge to the data we gather, “clean,” document, organize, and interpret. Salganik’s reframing of “designed” and “found” data in Bit by Bit: Social Research in the Digital Age is particularly important to this conversation (2017, 16). What would happen if scholars in Computational Social Science, Computational Humanities, Digital Humanities, Computational Linguistics and other fields broadened out to the widest possible purview and worked together to provide a new conceptual framework for how we might conceive of a dataset in machine learning? What if we could make it profoundly uncool to try to “solve” problems in other domains without consulting a domain expert?
Perhaps the best critique we might mount of the current state of machine learning is this: your method, results or performance are meaningless unless they help you to answer a question that someone outside of machine learning (and those who seek to monetize and promote it) cares about. Discovery and new knowledge in the domain should be the aim, not speed or performance or profit. As a scholar of poetry, I understand that genres are socially constructed–“modes of recognition instantiated in discourse” (2), Gitelman influentially wrote in 2014. A “ballad” in the eighteenth century printed on a broadside is an entirely different thing than Journey's 1995 “Open Arms.” We call them the same thing, but the historical and media contexts matter.
Like the benchmarks discussed by Raji, Bender et al., the original “ballad” is apocryphal – it is a cultural category that is only instantiated by association. Grover recognizes that things are bigger than he is in relation to his own size, but another room in the house he visits has a lot of small things–is he the big thing? What makes a thing big or small, categorizable in one way or another, is not only its immediate context (what is around it–collocation or topic models attempt to solve for this) but also its much broader social context (Bender at one point interjected “I’m a computational linguist and a socio-linguist”).
Bender used Meg Mitchell’s “black sheep” example to clarify this point: the modifier is required because it’s the exception to sheep; humans generally know that sheep are white and fluffy, but NLP logic does not, nor does it understand that the exception has larger cultural meaning. And, as we now well know (or should know, since scholars such as Benjamin, Meredith Broussard, and Noble have told us time and time again), many of the categories that are used by machines to classify are justified by those who built the algorithms because they say that the size of the data justifies the output – “hoovering” all of what the internet has available as an example of language or image as the background against which to measure what appear to be claims about actual culture – culture outside the door, the whole wide world Grover thinks he is in the museum to learn about.
But Grover’s museum isn’t actually the “whole wide world.” And like that museum, the internet is a closed system. Its size–the sheer amount of words and images–are not organized in a way that makes it a “sample” against which to measure anything. And I can see how referring to a dataset (like WordNet or ImageNet) rather than “everything in the whole wide world” makes it appear is if that dataset is smaller and more curated; makes it seem as if the scholars who are chasing faster, more accurate results aren’t making the same mistakes. But in actuality, these datasets are subject to bias and omission and inevitably replicate the harm that Benjamin, Broussard, Gebru, and Noble, among others, have cautioned against. Do we know enough about the contents of these data sets and how they were created? Are they small enough? Known enough? In upholding these datasets as valid constructs for testing progress (SOTA-chasing) – they have become their own closed system with the same unexamined problems.
Conclusion: Listen, I have an idea
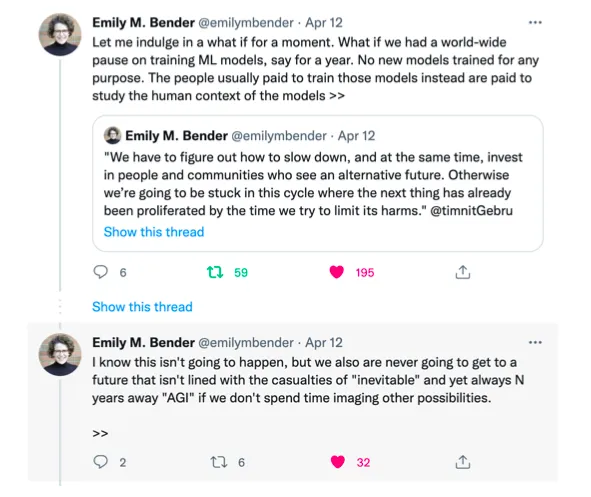
Note: The quotation in the quoted tweet (“We have to figure out…”) is from “Timnit Gebru is Building a Slow AI Movement”.
We don’t agree about language or meaning. Not in linguistics, not in literary studies, not in computational social sciences. And we don’t agree about data or datasets, but even if we don’t agree, we are eager to talk about the disagreement. Talking about disagreement and “harm” is not “soft data science” as it has been dismissively called; or rather the “soft skills” of critical thinking and effective communication in the data science domain should be front and center, rather than an afterthought. We must slow down to correct for the grave mistakes of an addiction to innovation – at the expense of interdisciplinary inquiry and collaboration. And the lack of response from the majority of the Machine Learning community, and the foundations and industries that support it, seem to resemble Grover’s own advice in another well-known children’s book There’s a Monster at the End of This Book: leverage Data Science to advance innovation, but let’s maybe not talk about what harm it might cause.
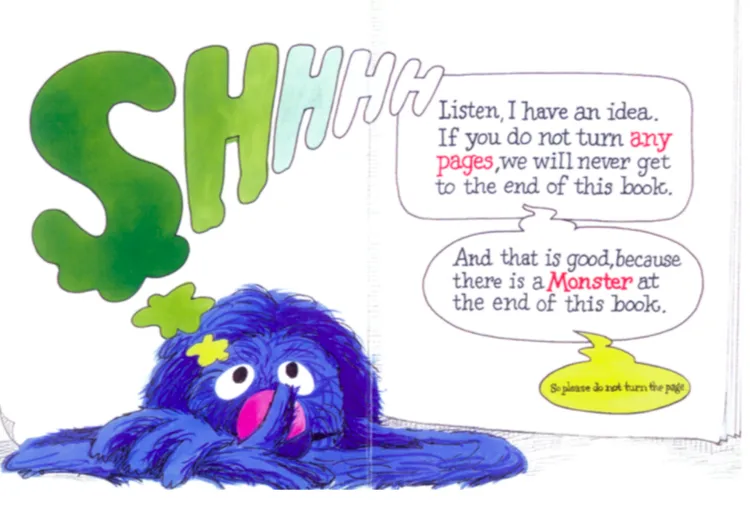
We can build infrastructures to try to mitigate the harm, but unless we work together to do so – across disciplines and subdisciplines and field divisions and academia and industry and journalism, our cautions will be dismissed – much as Gebru was dismissed by the company that hired her to co-lead its ethics division.
We know what happens at the end of the book already. It’s a monster, but it’s also us. The imperative research question for those of us who are not directly working in machine learning is how we can unify behind Bender and Gebru and other critics of AI so that we can all turn the page together.
With thanks for discussion with and edits from Sierra Eckert, Natalia Ermolaev, Lauren Goodlad, Rebecca Koeser, Emily McGinn and Kristin Rose.