End-of-Semester Reflections: Humanities Data Teaching Fellows
26 December 2021
The cohort of three graduate students developed modules for the undergraduate course, Introduction to Data Science.

Last May, I interviewed three current Humanities Data Teaching Fellows—Akrish Adhikari (G4, French and Italian), Gyoonho Kong (G5, German), and Daniel Persia (G2, Spanish and Portuguese)—about their expectations for the fellowship, which included working with the Center for Digital Humanities and the Center for Statistics and Machine Learning to develop modules for the undergraduate course “Introduction to Data Science” (SML 201).
At the end of the fall 2021 semester, I interviewed each of them to learn more about what their fellowships have been like.
The interviews have been lightly edited.
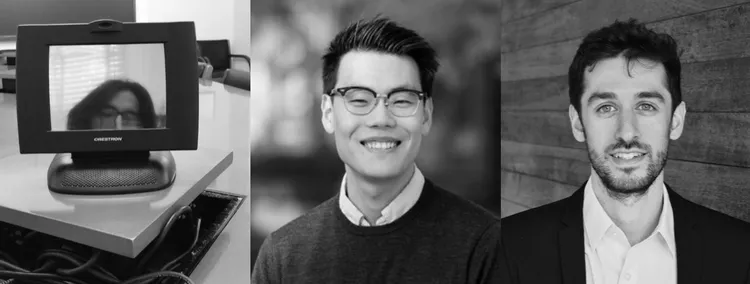
The 2021 cohort of Humanities Data Teaching Fellows (left to right): Akrish Adhikari, Gyoonho Kong, and Daniel Persia.
What did you develop for your SML 201 teaching module?
Gyoonho: I am planning to teach how to use the humanities dataset (in my specific case, German play data from 1700s up to the World War II era) to study data science, and specifically to learn the programming language, R. I will be teaching [the students] how to use functions including group_by(), factor(), filter(), summarize() etc., and using these functions to clean the play text data, and extract meaningful information out of the text by doing so.
Daniel: The module I developed is based on the Translation Database, an open-source project that tracks all fiction, poetry, children’s books, and nonfiction translated into English and published in the United States since 2008. The project was started by the literary hub Three Percent—in collaboration with Open Letter Books at the University of Rochester—and is now hosted by Publishers Weekly. The idea of the module is to generate and explore a range of questions about who gets translated and who does the translating in the contemporary literary sphere, considering country of origin, language, and author and translator gender, among other variables. Around 144 countries and 84 different languages are represented. Students learn how to manipulate data frames in R to better organize and visualize the data, responding to a series of preset questions while also formulating hypotheses of their own. Ethical questions about identity, representation, and data collection are embedded throughout.
Akrish: The module I developed allows students to visualize the emergence of the novel as we know it today (a literary form with chapters, a third-person or first-person narrator, etc.). The goal is to instruct students to make visualizations in R, while teaching them that the novel is a historical form. Questions the module encourages them to ask include: When did chapters become popular? And what is the relationship between the popularities of chapters and the third-person narrator choice—are they correlated? And so on.

A bar graph from Akrish’s work shows when chapters emerged in eighteenth-century France. “Livres” refers to “books” as the organizing feature of novels, while “parties” refers to “parts.” This module and its data are based on Nicholas Paige’s Technologies of the Novel: Quantitative Data and the Evolution of Literary Systems (Cambridge UP, 2020).
Besides creating a course module, what other projects did you work on during your time as a fellow—either as part of the fellowship program or outside of it?
Daniel: Creating the course module was the final, culminating moment of the Humanities Data Teaching Fellowship. Most of our time was spent working through the SML 201 curriculum, getting to know the ins and outs of the course and how our research questions might extend or deepen student learning. We also held regular meetings with members of the CDH to discuss new developments in digital humanities, such as data visualization and cultural analytics.
Gyoonho: I am currently working on my small private program called One-Card in Java, which will be a replication of a card game that I used to play in Korea with my family and friends. Of course, I am also working on my dissertation which is on video games and specifically representation of Germany in video games.
Akrish: I just focused on my dissertation.
What has surprised you about this experience?
Akrish: What surprised me is how challenging it can be to “insert” a humanities theme into an existing teaching plan in data science, even into a relatively rudimentary topic like data visualization. The dataset I chose did not necessarily lend itself to this visualization module, and this is something I only figured out while developing it. What this means is that I had to strike an uneasy balance between retaining the technical competencies the module previously covered, and introducing the humanistic context.
Gyoonho: I was surprised to see that I can visualize the humanities data using different graphs to see correlations between characters / words spoken / meaningful speech acts, etc., which allows us to check whether the intuitions and insights we develop about the text we research and read are correct.
Daniel: I was constantly reminded of how much overlap there is between data science and learning a second language. As a Spanish and Portuguese instructor, I’m always thinking about how to connect with students not only in the arts and humanities, but also in the natural and social sciences. And the thought process really isn’t all that different; there are certain grammars and structures that allow us to translate our thoughts into action, but we always need some idea of what we’re asking for or trying to say before we start to formulate the language.
How has this experience changed your own approach to research, teaching, or experiential learning?
Gyoonho: It allowed me to think in a more systematic manner (i.e., think about the texts from a more analytical perspective, rather than just approaching them with vague feeling or intuition about the meaning of the text), and to think about the quantifiable aspect of the text before delving into reading and researching process.
Akrish: It has motivated me into pursuing the computational humanities and its history more seriously. I’ve come across some astonishing work through this fellowship, especially Richard Jean So’s work. I am currently working to acquire a background in the field, which surprisingly stretches back to the nineteenth century, especially with techniques such as stylometry. I am also planning to take a class in natural language processing, which will build on my experience in this fellowship as well as prior coursework in machine learning.
Daniel: Collaborating not only with fellow humanities scholars but also with researchers and teachers in other divisions has been incredibly productive. I think we’re trained to see in a particular way in the humanities, and so when we step into the world of data science and suddenly we’re asked to see differently, that’s a really useful exercise. It’s about changing vantage point, approaching through a different lens. And that can lead to newer, bigger, more meaningful questions.
Do you have any advice for people who are interested in pursuing a Humanities Data Teaching Fellowship or similar program?
Akrish: I would make sure not to get behind on any of the assignments we do as part of the fellowship. It’s so easy to put things off, but the material in this class is undergraduate level: what this means is that there are several discrete topics with many small assignments, which graduate students, especially in the humanities, are not in the habit of doing.
Gyoonho: I would say—it would be good to really know the data set that you are proposing to use for developing the module, and that it would be really good to have some prior programming experience. Also, I would also suggest you start small and very realistic with the goals of your module, rather than planning to teach big and complicated concepts.
Daniel: The Humanities Data Teaching Fellowship has been an incredible experience. I now feel confident organizing and visualizing data in R, and I have a sense of how to orient learners quickly to the R environment. I have a broader sense of discussions and debates in the field of digital humanities, as well as an inside view of how curriculum is developed in an introductory data science course. For those less familiar or not at all familiar with the programming language being used, the demand might seem higher upfront, as you work your way through the course material. Scope is also key; it’s tempting to think big, but when you’re looking to teach a concrete set of skills, sometimes paring things down can be helpful. Having that conversation early on, when you’re still building out your vision for the module, will pay off in the long run.
The Humanities Data Teaching Fellowships are supported by a Magic Grant from the Humanities Council.